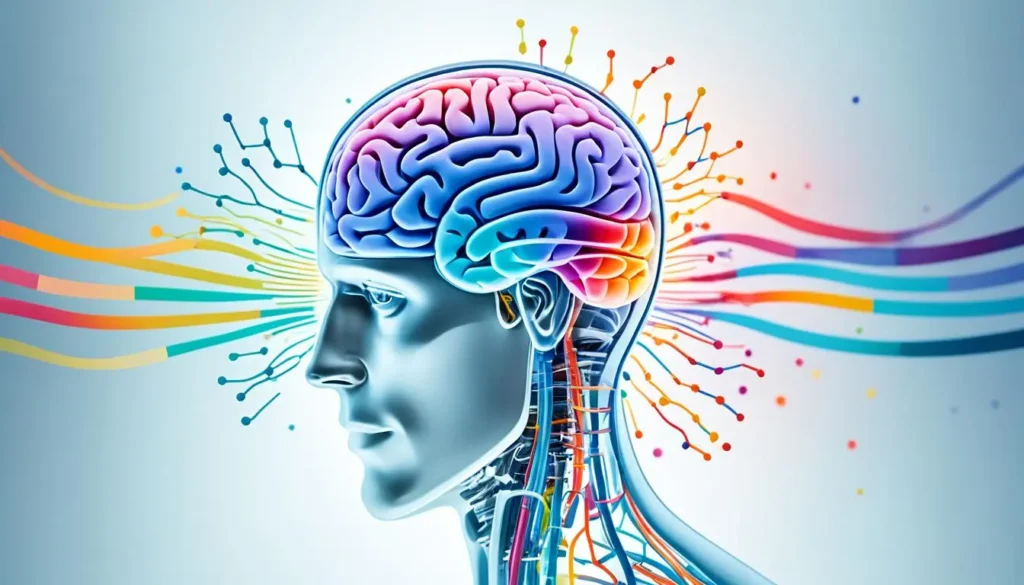
AI enhancing radiology reports accuracy is changing the medical imaging field fast. Thanks to artificial intelligence (AI), healthcare improves by helping doctors give more precise and efficient diagnoses. It also makes treatment plans more tailored to each patient.
Deep learning algorithms in medical imaging can spot problems and diseases faster and more accurately than before. This means doctors can make better diagnoses, treatments work faster, and patients get better care.
The market for medical imaging is set to jump from $31.9 billion in 2023 to $45.8 billion by 2030. This growth is thanks to more doctors using AI technology. AI helps make diagnoses more accurate by spotting patterns and anomalies quickly. It also cuts down on mistakes and makes radiologists better at their jobs.
Table of Contents
Key Takeaways
- AI technology in medical imaging can reduce the time for diagnosing stroke cases, resulting in faster intervention.
- AI-powered medical imaging can achieve an accuracy rate of 98.56% in brain tumor classification based on MRI-scanned images.
- AI algorithms can achieve diagnostic accuracy comparable to experienced radiologists in detecting breast cancer.
- AI integration in radiology reduces the time required for image interpretation, leading to quicker treatment decisions and improved patient outcomes.
- AI technologies can automatically segment organs, tumors, or lesions in medical images, enhancing radiologists’ efficiency in identifying key structures.
The Role of AI in Enhancing Radiology
AI is changing the game in radiology, bringing big improvements. It uses advanced algorithms to look at medical images fast and accurately. These algorithms spot patterns and changes that might escape the human eye.
AI can quickly find subtle changes in tissues using X-rays, MRIs, and CT scans. This is key for catching diseases early. It’s a big step up from the old days when radiologists had to rely on their eyes alone.
AI and Medical Imaging
AI in medical imaging brings many benefits. It does 3D imaging and reconstruction, makes reports automatically, and uses predictive analytics. It also improves image quality, plans treatments tailored to each patient, and uses radiomics to get detailed data from images.
It also helps with real-time monitoring and cuts down on mistakes in diagnoses. These changes make diagnosing diseases more accurate, make work easier, and help patients get better care.
Benefits of AI in Medical Imaging
- 3D imaging and reconstruction
- Automated reporting
- Predictive analytics
- Enhanced image quality
- Personalized treatment planning
- Radiomics (extracting high-dimensional data from images)
- Real-time monitoring
- Reduction of false positives and negatives
A study by Aerts et al. (2016) showed how radiomics could change medical imaging for the better. Radiomics is about getting detailed info from medical images. Deep learning, as noted by Litjens et al. (2017), is becoming key in analyzing medical images, making things more automated.
Study | Key Findings |
---|---|
Aerts et al. (2016) | Radiomic-based phenotyping in precision medicine has the potential to transform medical imaging. |
Kumar et al. (2012) | Radiomics involves the extraction of advanced features from medical images to provide more information. |
Litjens et al. (2017) | Deep learning methods are being widely used in medical image analysis, indicating a shift towards more automated processes. |
These AI advances in medical imaging could change radiology for the better. They promise to make diagnoses more accurate, streamline work, and improve patient care.
AI Enhancing Radiology Reports Accuracy
Artificial intelligence (AI) is changing the game in radiology, especially in cancer detection. It uses advanced machine learning to make tumor classification more accurate and efficient. This helps doctors tell apart benign and malignant tumors with great precision.
AI Cancer Detection
AI tools like convolutional neural networks (CNNs), support vector machines (SVMs), and random forests lead this change. They look through lots of medical data, including scans and patient histories. This helps them spot patterns that might mean a tumor is cancerous.
Adding AI to radiology work can make finding cancer more accurate. Studies show AI can beat even top radiologists in some tasks. For example, GPT-4 was 83% accurate in checking radiology reports, beating many doctors.
Commonly Utilized Models
- Convolutional Neural Networks (CNNs): These models are great at recognizing images and classifying them. They work well with medical scans like X-rays and MRIs.
- Support Vector Machines (SVMs): This algorithm is good at telling apart benign and malignant tumors. It helps doctors make reliable diagnoses.
- Random Forests: This method uses many decision trees to get better accuracy and avoid mistakes. It’s a strong tool for classifying tumors.
Using these AI models in radiology can make things run smoother, cut down on mistakes, and help patients by finding cancer earlier and making better treatment plans.
“AI in radiology can streamline workflow, shorten reading time, and improve diagnostic accuracy.”
Impact of AI on Radiologists
AI is not here to replace radiologists. Instead, it helps them make better and faster diagnoses. It also cuts down their work and mental load. AI in radiology brings many benefits, like ongoing learning, better workflow, and teamwork with other health experts.
AI is great at analyzing images and spotting patterns. This helps catch diseases early that are hard to see by hand. By doing routine tasks, AI makes radiologists’ work easier and faster. This makes healthcare work more efficient.
AI also helps radiologists work better with other health teams. It connects with systems like PACS and EHR. This keeps radiologists key in patient care. AI boosts their skills and helps them make better decisions.
Studies show AI cuts down reading time, especially when there’s no issue found. AI helps radiologists spot tiny details better. This leads to more accurate diagnoses and better patient care.
“AI algorithms in radiology excel in automated image analysis and pattern recognition, facilitating the early detection of pathologies that might be challenging to identify through traditional methods.”
As AI use grows in radiology, radiologists can use it to get better at their jobs. They can learn more and work better with AI systems. This helps them improve their skills, make their work smoother, and give patients better care.
Challenges and Pitfalls in AI Adoption
Artificial intelligence (AI) has shown promise in tasks like image segmentation and anomaly detection. Yet, its use in radiology has faced hurdles. The FDA approved computer-aided diagnosis (CAD) tools in 1998, but they didn’t catch on right away. Less than 5% of radiologists used them at first.
Studies found CAD didn’t make mammography more accurate. Instead, it led to more false positives. This was a setback.
AI tools struggle to use contextual info, which is key in medical imaging. They also find it hard with images of varying quality. This is because medical images can differ greatly due to different imaging systems and settings.
Pitfalls in Practice
AI development has often been driven by tech advances rather than clinical needs. This has led to a gap between AI’s capabilities and what radiologists really need in their work.
Need to Refocus
By focusing AI on different parts of radiology and on reducing medical errors, we can make radiology more efficient. This could boost the use and effectiveness of AI in healthcare. Integrating AI tools well can make imaging services better and faster, improving patient care.
AI can also help in creating new knowledge and supporting personalized medicine. For example, it can combine diagnostic imaging, clinical pathology, radiomics, and genomics for quicker, more accurate diagnoses.
“Refocusing AI on different aspects of the radiology workflow and medical error reduction can create new efficiencies in the practice of radiology, potentially increasing the clinical utility and adoption of AI.”
The main hurdles in using AI in radiology include:
- Low initial adoption rates of computer-aided diagnosis (CAD) tools despite FDA approval
- CAD tools not improving diagnostic accuracy and instead increasing false-positive rates
- Difficulty for AI tools to incorporate contextual information about patient conditions
- Struggles to deal with varying image qualities due to diverse imaging systems
- Technological push rather than being outcomes-driven by clinical needs
To overcome these issues, researchers recommend:
- Refocusing AI on different aspects of the radiology workflow to create new efficiencies
- Carefully integrating various AI tools to make the imaging service more effective and timely
- Utilizing AI to create new knowledge and support personalized medicine through the integration of diagnostic imaging, clinical pathology, radiomics, and genomics
By tackling these challenges and focusing AI development, we can greatly improve its use in radiology. This will lead to better patient care and outcomes.
Conclusion
AI is changing how we look at medical images, making diagnoses more accurate and efficient. It uses advanced algorithms to spot things in images that we might miss. This leads to catching diseases earlier and tailoring treatments to each patient.
Even though there are challenges, focusing on the whole radiology process can help unlock AI’s full potential. This could lead to fewer mistakes and better patient care.
As AI gets better, it will be key in making healthcare more personal. It could make patients’ outcomes better, cut healthcare costs, and make things run smoother. This could lead to a healthcare system that works better for everyone.
The future of radiology looks bright with AI. It’s set to bring new ways of diagnosing and treating patients. This could start a new chapter in healthcare, making it more precise and tailored to each person.
FAQ
What is the role of AI in enhancing radiology reports accuracy?
AI is changing healthcare by making diagnoses more accurate and efficient. It uses deep learning to spot problems and diseases faster than before.
What are the benefits of AI in medical imaging?
AI in medical imaging brings many advantages. It does 3D imaging and reconstructs images. It also automates reporting and uses predictive analytics.
It improves image quality and helps plan treatments. It uses radiomics to get detailed data from images. It also monitors patients in real-time and cuts down on mistakes.
How is AI being utilized in cancer detection?
AI is a key tool in fighting cancer. It can tell apart benign and cancerous tumors. By looking at lots of medical data, AI spots and classifies tumors very accurately.
What are some commonly utilized AI models in radiology?
In radiology, AI models like CNNs are great for recognizing images. SVMs are good for classifying things. Random forests use many decision trees to get better at predicting outcomes and avoid overfitting.
How does AI impact the work of radiologists?
AI doesn’t replace radiologists; it helps them work better. It makes diagnoses more accurate and quick. This reduces their workload and helps them learn more.
AI also improves how radiologists work together with others in healthcare. It makes their work more efficient.
What are some of the challenges and pitfalls in the adoption of AI in radiology?
Even though AI tools got FDA approval in 1998, they didn’t catch on fast. Early studies showed they didn’t make mammography better and raised false alarms.
AI can’t yet understand the full story behind a patient’s health. It also has trouble with images of different quality. This is because images can vary a lot due to different equipment and settings.