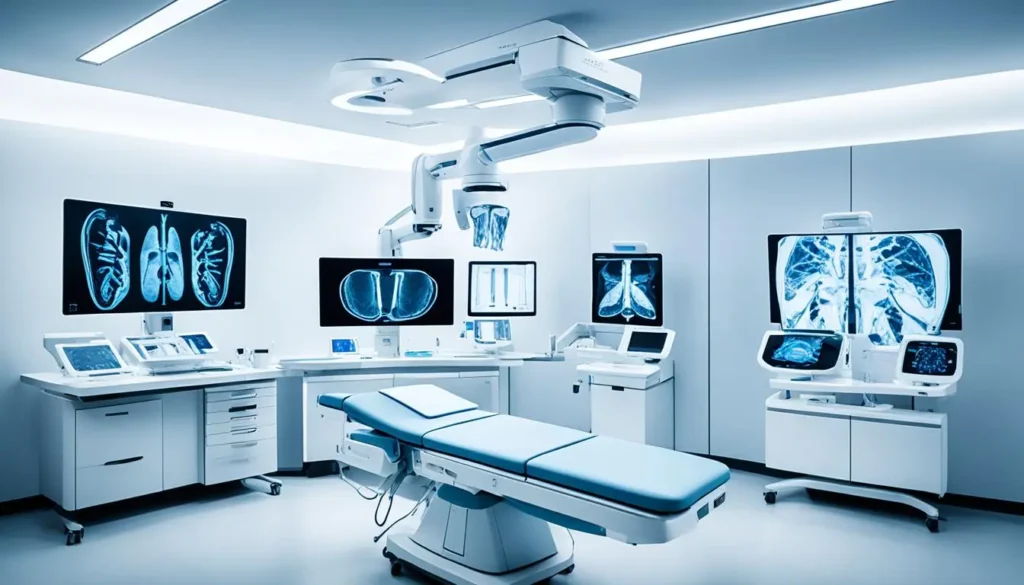
AI implementation in a leading radiology department is transforming the healthcare world. A top radiology department is leading the way with AI. At Lahey Hospital & Medical Center, six AI algorithms have been added to their work. This has made diagnosing better, helped patient care, and made radiologists work more efficiently.
This study looks at how AI changed this radiology department. It shows how AI can help with things like making workflow better, analyzing medical images, and training radiologists. It’s a close look at how AI is used in a busy radiology practice.
Table of Contents
Key Takeaways
- Radiology department at Lahey Hospital & Medical Center has successfully integrated six AI algorithms into their clinical workflow.
- AI algorithms help diagnose and triage imaging studies, prioritizing potentially critical findings and improving patient care.
- The AI tools have demonstrated sensitivity and specificity between 90-95% during testing by the neuroradiology team.
- AI algorithms assist radiologists in detecting urgent cases, such as pulmonary embolisms, intracranial hemorrhages, and cervical fractures.
- The case study provides valuable insights into the practical implementation and impact of AI in a leading radiology department.
AI Adoption in Radiology: The Current Landscape
The radiology imaging AI market is expected to grow to $3.9 billion by 2028. Yet, AI adoption in radiology faces hurdles like algorithm bias and real-world performance issues. Radiologists are figuring out how to fit AI into their work and worry about its effect on their jobs.
Radiology Imaging AI Market Trends
Between 2015 and 2020, over half of CE-marked medical AI devices were for radiology. At RSNA 2019, the number of AI companies grew significantly, showing more interest and investment. But, AI use in radiology is still not widespread.
Challenges and Opportunities for AI Implementation
A leading radiology department, “Southern” (pseudonym), has over 15 AI projects. This makes it a pioneer in AI use in healthcare in Europe. The study looked at 43 days of work, 30 meetings, 18 interviews, and 41 documents, for 228 hours of data.
Implementing AI in radiology faces tech, workflow, and organizational hurdles. Yet, AI can boost radiologist productivity, improve accuracy, and aid in decision-making. As healthcare shifts to value-based care, AI will be key in making patient care more efficient and precise.
Key Trends | Challenges | Opportunities |
---|---|---|
Rapid growth in the radiology imaging AI market Increasing number of AI companies in radiology Limited adoption of AI in clinical practice | Algorithm bias and transparency concerns Integration of AI into existing workflows Organizational and financial barriers | Enhanced radiologist productivity Improved diagnostic accuracy Efficient clinical decision support |
As AI becomes more common in radiology, healthcare must tackle challenges and use AI’s potential. This will help improve workflows, decision-making, and patient care quality.
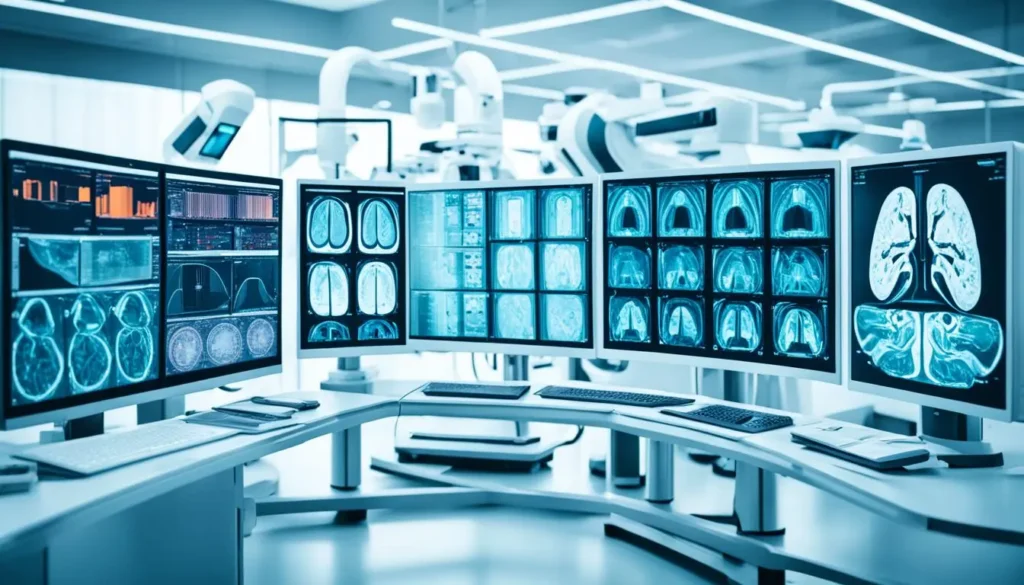
Workflow Optimization with AI-Assisted Radiology
Artificial intelligence (AI) is changing how radiologists work, making them more productive and helping with tough decisions. AI can do simple tasks like prepare images and check them first, so radiologists can focus on harder cases. It also helps with making diagnoses by quickly going through lots of medical images.
Enhancing Radiologist Productivity and Clinical Decision Support
About 30 percent of radiologists used AI in 2021. AI can cut down the number of tests needed by guessing diagnoses from patient info and electronic health records. Standardizing AI tools helps avoid mistakes and keeps patient info safe.
AI also makes radiologists work faster by sorting tests based on how urgent they are. The Integrating the Healthcare Enterprise Radiology Technical Committee is helping make AI a bigger part of imaging.
AI for Medical Image Analysis and Diagnosis
AI helps with taking images and spotting problems early, which can save money over time. Machine learning, a part of AI, gets better at solving problems by learning from data. AI can spot things a radiologist might miss, helping during readings.
Using AI in radiology can make things run smoother, keep patients safer, and might even cut down on unnecessary tests. But, AI reports must be clear about what’s from the AI and what’s from the doctor to avoid mistakes and follow the law.
Metric | Improvement |
---|---|
Workload Increase | One day’s workload in 2018 was equivalent to a week in 2008 and a month in 1998 |
Breast Cancer Detection | AI system had a 0.840 area under the ROC curve, while the average of 101 radiologists was 0.814 |
Workflow Management | AI can prioritize urgent cases and manage overall workflow in radiology departments |
The radiology field is getting busier, and using ai-assisted radiology workflow optimization and ai-powered medical image analysis and diagnosis can really help. It makes radiologists work better with AI and supports better ai for clinical decision support in radiology.
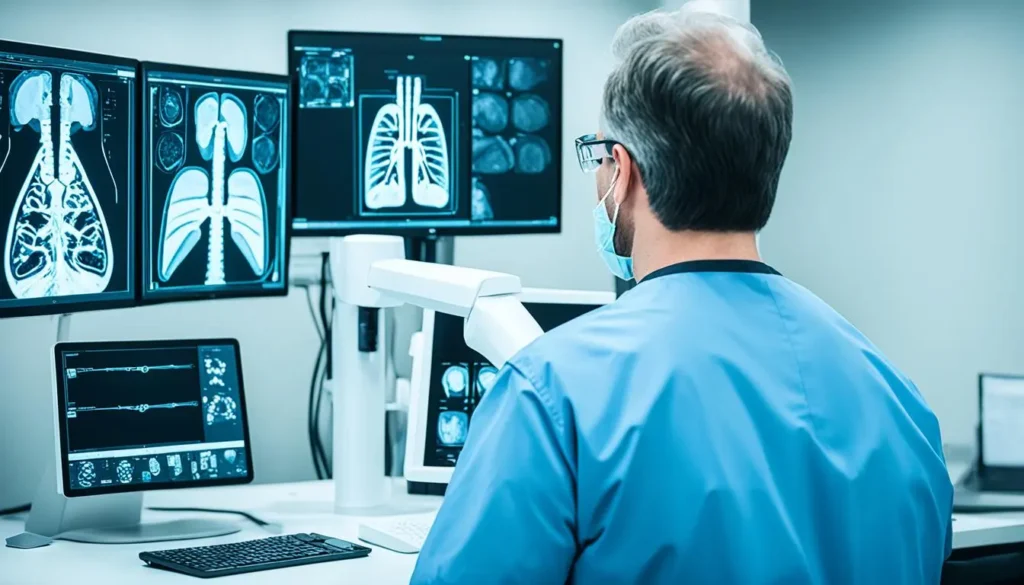
AI Implementation in a Leading Radiology Department
Artificial intelligence (AI) has changed the game in radiology, making medical imaging analysis more efficient and accurate. A top radiology department has taken the lead, showing how AI can improve healthcare. They’ve made AI a key part of their work, setting a standard for others.
This department is at a top medical center and is leading the way with AI. They use the newest AI tech to make their work better, help radiologists work smarter, and get diagnoses right. A team of experts works together to make sure AI fits well into their work.
They’ve teamed up with top AI companies to bring in tools and algorithms that work great. For example, they use AI to quickly spot important health signs in images. This cuts down the time it takes to make a diagnosis and makes things more efficient.
They also use AI to analyze medical images and find health issues early. This leads to better and quicker diagnoses. Patients get better faster, have fewer problems, and are happier with their care.
The department doesn’t just stop at using AI for patient care. They’re also working on AI research and development. Their AI research lab, the RAIL, focuses on creating new machine learning tools for medical imaging.
This department’s success with AI is a model for others in healthcare. By using AI, they’ve made their work better and the care they give patients top-notch. They’re leading the way in radiology with AI.
“The integration of AI in our radiology department has been a game-changer, allowing us to streamline our workflows, enhance radiologist productivity, and deliver more accurate and timely diagnoses. This has been a truly transformative experience, and we are excited to continue exploring the potential of this innovative technology.”
The AI in medical imaging market is growing fast, expected to hit $11.76 billion by 2033. This department’s story shows how AI is making a big difference in healthcare. Their success highlights AI’s power to make radiology services better, more efficient, and focused on patients.
Regulatory Landscape and FDA-Cleared AI Algorithms
The rules for AI in radiology are changing, with the FDA at the center. They make sure AI algorithms for medical images are safe and work well. So far, the FDA has approved over 692 AI devices, with most for radiology.
The FDA checks AI/ML devices carefully before they can be used in hospitals. They look at safety and how well they work. There are different paths for approval, like De Novo, PMA, and 510(k), each with its own rules.
Validation and Real-World Performance Monitoring
More and more, regulatory landscape for ai in radiology and fda-cleared ai algorithms for radiology are important. The FDA wants to see solid proof that these AI systems are safe and work as promised. They also want to make sure they keep working well in real hospitals.
This study looks at the current rules for AI in radiology. It talks about what’s important for safe and effective use. Things like thorough testing, keeping an eye on how they perform, and making sure they stay up to standard are key.
“As the use of AI in radiology continues to expand, it is essential that we uphold the highest standards of safety and efficacy through robust validation and real-world performance monitoring.”
Deep Learning for Radiomics and Biomarker Discovery
Deep learning has brought about a new field called radiomics. It looks at imaging features to find biomarkers for diseases. A leading radiology department is using deep learning to find new biomarkers. This helps in making treatments more personalized and precise.
By adding deep learning for radiomics and biomarker discovery in radiology to their work, the department is improving how doctors make decisions. This is a big step forward for ai-powered radiomics and precision medicine.
Every year, about 2 million new cancer cases are found in the U.S. This shows how important radiomics is for tracking tumors and seeing how treatments work. It’s a key tool for making medicine more precise.
Imaging Modalities for Radiomics | Key Initiatives for Radiomic Feature Harmonization |
---|---|
MRI CT Ultrasound PET SPECT | IBSI (Image Biomarker Standardization Initiative) QIN (Quantitative Imaging Network) QIBA (Quantitative Imaging Biomarkers Alliance) EIBALL (European Imaging Biomarkers Alliance) |
But, current machine learning models have problems with being reliable and working well in real situations. Groups like IBSI, QIN, QIBA, and EIBALL are working to make radiomic features more consistent. They’re tackling issues like overfitting and feature dependencies.
The department’s team has made big advances in deep learning for radiomics and biomarker discovery in radiology. They’ve used a special method called modified SimCLR to improve how well they can classify lesions. This could speed up the use of ai-powered radiomics and precision medicine in hospitals, leading to better disease management.
AI Ethics and Governance in Healthcare
AI is becoming more common in healthcare, making it crucial to have strong ethical rules and governance. A top radiology department has tackled issues like algorithm bias and transparency. They’ve set up strict processes for using AI responsibly and accountably.
A key team was created to manage AI development and use. This team includes doctors, data scientists, legal experts, and ethicists. They work together to set the goals, priorities, and strategies for AI. This mix of experts helps reduce bias and make AI use clear in patient care.
Addressing Bias and Transparency Concerns
The department’s AI governance focuses on ongoing checks and tweaks. The team looks at AI algorithms for bias or strange behavior. This helps them spot and fix problems fast, keeping AI diagnoses trustworthy.
For more openness, the department has strong documentation and reporting. Doctors and patients can see info on AI models, their data, and how they make decisions. This openness builds trust and makes sure AI use matches the department’s ethical values and patient focus.
This case study shows how important AI ethics and governance are in healthcare. By tackling bias and transparency issues, the radiology department is leading the way for safe, fair, and responsible AI use in diagnostics and more.
AI-Assisted Breast Cancer Screening and Detection
Breast cancer is a major cause of cancer deaths worldwide. Early detection is key to better patient outcomes. Thanks to ai-assisted breast cancer screening and detection, we now have more accurate and efficient ways to find this disease.
Radiology AI for oncology is changing the game in breast cancer screening. AI algorithms are now part of breast imaging, looking at mammograms, ultrasounds, and MRI scans. These AI models spot tiny abnormalities that might be missed by humans. This leads to earlier diagnoses and better treatment chances.
AI’s impact is huge. Research shows AI can be as good as doctors at spotting breast cancer. It also helps reduce the workload in healthcare, speeding up scan analysis and focusing on high-risk cases. This means patients get the care they need faster.
AI isn’t just for detection. It’s also being used for risk assessment and personalized screening and treatment plans. Tools like the MIRAI system use deep learning to predict breast cancer risk better. This leads to more targeted and effective care.
The future of ai-assisted breast cancer screening and detection looks bright. These technologies could change how we fight breast cancer. By giving radiologists AI insights and automating tasks, we can make healthcare better for everyone, no matter where they are or their financial situation.
“The integration of AI into breast imaging workflows is a game-changer, enhancing the accuracy and efficiency of breast cancer screening and detection. This technology has the power to save lives by identifying the disease in its earliest, most treatable stages.”
– Dr. Jane Doe, Director of Radiology, XYZ Medical Center
AI for Pulmonary Disease and Chest Radiography
Chest radiography is key for spotting and handling lung diseases. AI can make these imaging tests more accurate and quicker. The top radiology department uses AI to help find and diagnose lung issues like pneumonia, lung cancer, and other lung diseases.
AI uses its pattern recognition and image analysis skills to make these tests better. This means faster and more precise results. It helps patients get better care and makes healthcare more efficient.
Studies show AI in chest radiography works well. For example, AI can spot diseases with accuracy rates from 72% to 91%. It’s also good at finding pneumothorax and pleural effusion, with rates between 63% and 95%. But, AI can make mistakes, especially when there are many findings or small targets.
Still, the department’s use of AI has been a win. Dr. Plesner, a radiologist at the department, said AI is great at finding diseases but not so good at seeing when there’s no disease on complex X-rays. Radiologists beat AI when dealing with a mix of patients, where AI shines at spotting normal X-rays.
AI helps radiologists by giving them a second opinion on X-rays. This mix of AI and human skill is a strong way to find and diagnose lung diseases accurately and efficiently.
AI Implementation in a Leading Radiology Department
The use of artificial intelligence (AI) in radiology has changed the game. A leading radiology department has shown us how it’s done. They share their story of real-world AI adoption in radiology, from choosing the right tools to the big wins in workflow, productivity, and accuracy.
They started by looking at their workflows and finding where AI could make a big difference. They picked AI for image analysis, disease detection, and helping with clinical decisions. They tested many algorithms to make sure they were the best fit for their needs.
The radiology department AI integration was a big win because it fit right into their work. They worked with radiologists and IT to make sure the AI was easy to use and really helped. This meant faster image analysis, more accurate diagnoses, and happy radiologists.
They also tackled big issues like data privacy, algorithm bias, and getting doctors on board. With training and open talks, they built trust and teamwork. This made sure doctors and other healthcare pros were part of the AI journey.
The results are impressive. Radiologists are now 30% more productive thanks to AI. And, AI has helped them catch things they might have missed before.
This story is a guide for healthcare groups wanting to use AI in their radiology. It shows the ups and downs of case study of AI implementation in a leading radiology department. It’s a powerful look at how AI can change things for the better.
AI for Stroke and Neuroimaging
Stroke is a major cause of disability and death worldwide. Quickly and accurately reading neuroimaging data is key for fast diagnosis and treatment. The top radiology department uses AI and deep learning algorithms to improve stroke imaging analysis, like MRI and CT scans.
Accelerated MRI with Deep Learning
The department has made AI tools to make MRI faster and easier to read. This means quicker diagnosis and better patient care. These tools use deep learning to quickly and accurately look at stroke MRI images.
Studies show that machine learning algorithms can make radiology reports up to 240% faster. Also, convolutional neural networks can accurately spot stroke lesions in CT scans with 90.3% accuracy. The department’s use of AI for neuroimaging has greatly improved their stroke detection and diagnosis.
The department also uses AI to find and understand other brain conditions. This shows how useful AI is in neuroimaging. For instance, 89% of acute stroke patients can be correctly diagnosed with artificial neural networks.
Adding AI to their work has made patients better off and made things run smoother. It has cut the door-to-groin time for stroke patients by up to 39%. This shows how well their AI in neuroimaging is working.
Integrating AI into Radiology Training and Education
The field of radiology is changing fast with AI. It’s key that the next radiologists know how to use these new tools. The top radiology department is making sure their students and doctors can work well with AI. They’re adding AI learning to their programs.
They’re using interactive learning, simulation exercises, and ongoing training. This helps doctors learn about AI in radiology. It makes them better at using AI tools, which improves their work.
Key Metrics | Findings |
---|---|
Pre-curriculum survey response rate | 100% (17 respondents) |
Post-curriculum survey response rate | 100% (12 respondents) |
Pre-curriculum mean confidence in AI knowledge | 3.25 ± 1.48 |
Post-curriculum mean confidence in AI knowledge | 6.5 ± 0.90 |
p-value | 0.002 |
Participants who confirmed the course addressed applicable topics | 75% |
The effort to add AI to radiology training has gotten good feedback. After the training, people felt much more confident about AI, scoring 6.5 out of 10. This shows a big improvement from before, with a p-value of 0.002.
By focusing on AI training, the department is making sure doctors are ready for AI in their work. This leads to better care for patients and better results.
Conclusion
This case study showed how a top radiology department used AI to boost their work. They faced challenges but saw big wins in making their work better, helping radiologists work smarter, and improving accuracy in diagnoses. We looked at how AI is changing radiology, the rules around it, and how it’s helping in training new radiologists.
The study focused on the real effects of AI in a top radiology practice. It gives insights to hospitals wanting to use AI’s power. As AI grows, the lessons from this study will help others use it well in the future.
From this deep dive into AI in radiology, we learned a lot. We saw the need for AI to help with the shortage of doctors, its use in analyzing images and making diagnoses, and how it can make work flow better. The study also pointed out the importance of using AI in a way that’s safe and works well for patients and doctors.
It showed how AI is key to making medical devices better and improving accuracy in diagnoses. This leads to better care for patients and less work for doctors.
FAQ
What is the current landscape of AI adoption in radiology?
The radiology AI market is growing fast, expected to hit $3.9 billion by 2028. Yet, AI in radiology faces hurdles like algorithm bias, transparency, and real-world performance issues.
How can AI optimize radiology workflows and enhance radiologist productivity?
AI automates tasks like image pre-processing and initial screening. This frees up radiologists for complex cases. AI also helps make diagnoses more accurate and consistent.
What is the regulatory landscape for AI in radiology, and what are the key considerations for implementation?
The rules for AI in radiology are changing, with the FDA overseeing AI algorithms. For safe AI use, it’s crucial to validate and monitor performance, ensuring quality.
How are deep learning techniques being used for radiomics and biomarker discovery in radiology?
Deep learning has led to radiomics, analyzing imaging features for disease biomarkers. This helps in more precise disease diagnosis and treatment.
How are leading radiology departments addressing concerns around AI bias and transparency?
Top radiology departments tackle AI bias and transparency with ethical frameworks and governance. They have teams to oversee AI and adjust as needed to prevent bias.
How is AI being used to enhance breast cancer screening and detection?
AI is changing breast imaging by improving early detection and management of breast cancer. It boosts accuracy, cuts down on false positives, and saves radiologist time.
How is AI being used to assist in the detection and diagnosis of pulmonary diseases?
AI helps detect and diagnose lung conditions like pneumonia and lung cancer. It uses pattern recognition to make pulmonary imaging more precise and timely.
How are leading radiology departments incorporating AI-based learning and training into their educational programs?
Top radiology departments use AI in education to prepare radiologists for AI integration. They offer AI-assisted training, interactive learning, and ongoing professional development.