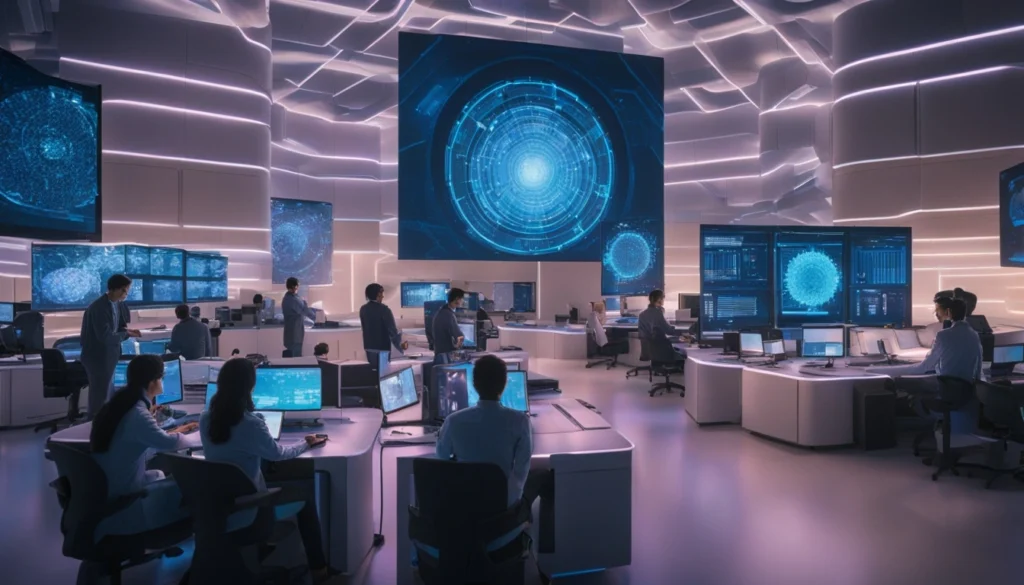
AI in Identifying New Drug Targets, Artificial intelligence (AI) is changing the game in pharmaceutical research. It’s not just speeding up the process. AI is opening new doors by finding new ways to treat diseases. This tech could be the key to discovering the next big breakthrough in medicine.
AI uses machine learning and deep learning to quickly go through huge amounts of data. This includes things like genes, proteins, and how our bodies work. By understanding these complex systems, scientists can find new targets for drugs. This could lead to better treatments for many diseases.
Table of Contents
Key Takeaways
- AI-driven methods, such as machine learning and deep learning, can accelerate the drug discovery process by quickly processing and analyzing large volumes of biological data.
- AI has the potential to uncover new therapeutic targets by providing a better understanding of the complex molecular mechanisms underlying diseases.
- Integrating multiomics data, including genomics, proteomics, and metabolomics, is crucial for AI-powered target identification and drug discovery.
- Network-based biology analysis algorithms and machine learning-based biology analysis algorithms play a vital role in identifying potential anticancer targets and novel drug candidates.
- The collaboration between pharmaceutical companies and AI firms highlights the industry’s interest in leveraging AI technologies for drug discovery and development.
Introduction to AI in Drug Discovery
The pharmaceutical industry is now more digital, creating lots of data. Artificial intelligence (AI) helps analyze this data. Tools like IBM Watson help with patient data and treatment plans. Machine learning (ML) and deep learning (DL) find new drugs and check their safety, making finding new medicines faster and cheaper.
AI helps by looking through huge lists of chemicals to find new medicines. It can tell which ones might work well and be safe. This has changed how new drugs are made, making it faster and more efficient.
The Role of AI in Drug Discovery
Machine learning (ML) and deep learning (DL) are key in finding new medicines. They help spot promising medicines, check if they target the right area, and make them better. This makes finding new medicines quicker and cheaper.
- AI can look through lots of chemicals to find new medicines and guess how they’ll work in the body.
- It can also look at patient data to suggest the best treatments, making medicine more personal.
- AI helps automate lab work, cutting down on mistakes and making results more reliable.
“AI has the potential to revolutionize drug discovery by accelerating the process and improving the quality of drug candidates.”
AI is becoming a big part of finding new medicines. The pharmaceutical industry is using it more to make the process better. As artificial intelligence gets better, it will likely make finding and developing new medicines even more efficient and effective.
Scope of AI in Target Identification
AI has a huge role in finding new drug targets. It can handle complex biological data from many sources like genomics, proteomics, and metabolomics. By combining these data, AI finds the complex links between biological parts. It spots important parts in these networks and finds potential targets for treatment.
Multiomics Data Integration
Algorithms for network-based biology have made it easier to use molecular data in genomics analysis. These methods gather data on how genes work together. They turn this data into clear biological processes. This helps find new targets for drugs.
- On average, it costs around USD $2.5 billion to see a drug to market, considering successful and unsuccessful trials in the pharmaceutical industry.
- AI technologies could generate “time and cost savings of at least 25–50%” up to the preclinical stage in drug discovery according to the BCG and Wellcome report.
- Availability of sufficient/high-quality data sets was the most common barrier to increasing the usage of AI tools in drug discovery, with 64% of respondents citing it as a significant roadblock.
- Limited data quality or availability can restrict the effectiveness of AI when screening large chemical libraries, potentially leading to missed opportunities for identifying promising targets and compounds.
AI uses multiomics data and network analysis to change how we find new drug targets. This could speed up drug discovery and increase success rates.
“90% of drugs fail during clinical trials, but 24 AI-discovered molecules have completed Phase I trials with a success rate of 80-90%, and 10 AI-discovered molecules had completed Phase II trials with a success rate of 40%.”
Companies like Insilico Medicine have already sold preclinical candidates found with AI to pharmaceutical companies for about half a billion dollars. This shows how powerful AI is in finding new drugs.
Network-based Biology Analysis Algorithms
Network-based biology analysis algorithms are key in finding new cancer targets for drugs. They look at biological networks from different angles. This helps them make accurate predictions by using each other’s strengths. These algorithms focus on sequence-similarity networks, genome networks, and gene family networks.
These tools are great at dealing with the complex nature of living systems. They show how genes, proteins, and metabolites interact with each other. Also, consensus clustering algorithms help group biological networks into parts with specific roles. This has led to the discovery of new cancer genes and potential treatments.
- Sequence-similarity networks: These look at how similar genes or proteins are. They help find new drug targets by showing functional links.
- Genome networks: These map out genetic interactions and how genes work together. They help find genes linked to diseases and potential drug targets.
- Gene family networks: These networks look at how genes in families relate to each other. They shed light on what genes do and their potential as targets for treatment.
- Consensus clustering algorithms: These break down biological networks into smaller groups. This helps find new cancer genes and potential treatments.
Using these algorithms has changed the drug discovery field a lot. Researchers now have a deep understanding of complex biological systems linked to diseases. These tools help scientists find new targets for drugs. This leads to better treatments and outcomes for patients.
“Network-based biology analysis algorithms have revolutionized the way we approach drug discovery, unlocking new possibilities for identifying effective cancer targets.”
AI in Identifying New Drug Targets
Machine learning (ML) is now a key tool for finding new drug targets. It uses algorithms like multilayer perceptron (MLP), recurrent neural networks (RNNs), and convolutional neural networks (CNNs). These algorithms can handle lots of complex data and find important patterns in biological networks.
ML helps with tasks like recognizing patterns, optimizing processes, and identifying controls. For instance, deep learning (DL) models are better at predicting how drugs work in the body than older ML methods. This is important for understanding drug properties.
Machine Learning Techniques
Using ML and DL, researchers can quickly find and check new drug targets for diseases. In the last five years, money spent on AI in drug discovery has grown a lot. It went from $2.4 billion in 2020 to over $5.2 billion in 2021.
AI has also sped up vaccine development. It can make vaccines in less than a year, which is much faster than before. This is important because making a drug can take 12 to 18 years and costs about $2.6 billion.
AI can also predict complex protein structures well, which helps in finding drug targets. But, only 11% of the human proteome has been studied with small molecule probes. This means about 33% is still not well understood.
Companies like Atomwise use AI to check over 100 million compounds every day. This helps predict if a medicine could work and cuts down on costs. Deep Genomics has a big database with predictions for over 300 million genetic variations. They focus on RNA therapies for specific genetic diseases.
There’s a lot of research on using AI in drug discovery. Many articles have been published in journals like Nature Communications and Expert Opinion on Drug Discovery. By using AI, researchers can find and validate new drug targets faster. This leads to better and more targeted treatments.
Applications of AI in Drug Discovery
Artificial intelligence (AI) has changed the way we find and develop new drugs. It helps in designing drugs, screening compounds, and improving them. AI uses complex models to quickly find and improve drug candidates. This could make it faster and more successful to bring new drugs to the market.
AI-Assisted Drug Design
AI helps design new drugs by predicting their properties, how well they work, and if they are safe. It looks at many possible compounds fast. This lets researchers focus on the best ones and cut down on tests.
AI-Powered Virtual Screening
AI can quickly go through huge lists of chemicals to find ones that fit well with target proteins. This speeds up finding new leads. Researchers can then focus on these promising molecules for more testing.
AI in Drug Repurposing
AI also helps in finding new uses for drugs already on the market. It looks at lots of data to find patterns that suggest a drug could be used for something else. This makes finding new uses for drugs faster.
AI in Clinical Trials
AI can make clinical trials better by finding the right patients, designing trials well, and keeping an eye on patients. It uses medical records to find the best patients. It also watches for problems and can change the trial as needed.
AI Application | Impact on Drug Discovery |
---|---|
Rational Drug Design | Predicts physicochemical properties, efficacy, and safety of drug candidates |
Virtual Screening | Rapidly evaluates large chemical libraries to identify promising lead compounds |
Drug Repurposing | Analyzes existing compounds to uncover new therapeutic indications |
Clinical Trials | Enhances patient recruitment, optimizes trial design, and improves monitoring |
AI is changing drug discovery for the better. It makes finding and improving new drugs faster and more efficient. This could lead to quicker and more successful drug development.
Challenges and Future Directions
The pharmaceutical industry is diving deep into AI for drug discovery. But, it’s facing big challenges. The main issue is the huge amount of data available for drug development. This data is complex and hard to manage with traditional tools.
That’s why advanced AI, like deep learning, is key to unlock the data’s potential. The virtual chemical space is huge, and finding the right drug candidates is tough. Overcoming these data challenges is vital for the future of AI in drug discovery.
There are also ethical concerns about using AI in this field. We need to make sure AI is transparent, accountable, and fair in its decisions.
Despite these hurdles, the future looks bright for AI in pharma. We’ll see progress in drug repurposing, patient-focused clinical trials, and global research collaboration. AI will work with new tech like digital twins and organ-on-a-chip to better understand patients and speed up personalized treatments.
To make AI work in drug discovery, we need a comprehensive approach. This means tackling technical, ethical, and regulatory issues. By working together and improving data quality, the pharma industry can use AI to open new doors in drug development and better patient care.
Key Challenges in AI-Powered Drug Discovery
- Handling the scale, growth, diversity, and uncertainty of drug development data
- Developing effective virtual screening methods to identify promising drug candidates
- Ensuring transparency, accountability, and fairness in the AI decision-making process
Future Directions for AI in Pharmaceutical Industry
- Advancements in deep learning and other advanced AI techniques to better manage complex datasets
- Integrating AI with emerging technologies, like digital twins and organ-on-a-chip, for personalized treatment development
- Exploring the potential of AI in drug repurposing and patient-centric clinical trials
- Fostering global collaboration and interdisciplinary research to drive innovation in AI-powered drug discovery
“The successful implementation of AI in drug discovery will require a multifaceted approach that addresses the technical, ethical, and regulatory hurdles.”
Conclusion
AI has changed the game in the pharmaceutical world, especially in finding new drugs. It uses machine learning and deep learning to quickly go through lots of complex data. This helps spot patterns and make predictions that speed up finding new medicines.
AI helps in many parts of making new drugs, like designing them, testing them, and improving them. It also helps in finding new uses for old drugs and making clinical trials better. This could save the US healthcare system up to $100 billion a year.
Companies like Atomwise, Insilico Medicine, and DeepMind are already using AI to predict how drugs work with the body. As AI and machine learning get better, they will keep changing how we make and test drugs. This will lead to cheaper, more effective treatments for many diseases.
FAQ
How can AI assist in the drug discovery and development process?
AI helps in many ways in drug discovery and development. It aids in designing drugs, screening them virtually, and improving leads. AI uses computational models to quickly predict how drugs work and their safety. This lets researchers focus on the best options.
AI also looks at old drugs for new uses. It makes clinical trials better by finding the right patients and improving trial designs. Plus, it tracks how patients do in trials.
How can AI help in identifying new drug targets?
AI is great at looking through complex biological data from many sources. It uses this data to find important connections in biological networks. This helps find new drug targets.
AI uses special algorithms to analyze these networks. These algorithms help make sense of large amounts of data. This makes finding new drug targets easier.
What are the key machine learning techniques used in drug target identification?
Machine learning is key in finding new drug targets. Techniques like multilayer perceptron networks and recurrent neural networks work well with complex data. They help spot patterns and relationships in biological networks.
These methods are used for many tasks, like recognizing patterns and optimizing processes. Deep learning models are especially good at predicting how drugs work and their safety.
What are the challenges in integrating AI in the pharmaceutical industry?
Integrating AI in pharma has big potential but faces challenges. Handling the vast amounts of data is one big issue. Traditional ML tools struggle with this.
There’s also a huge space of possible drugs to screen. Advanced AI, like deep learning, is needed to tackle this. Ethical concerns, like making sure AI is fair and transparent, also need to be addressed.