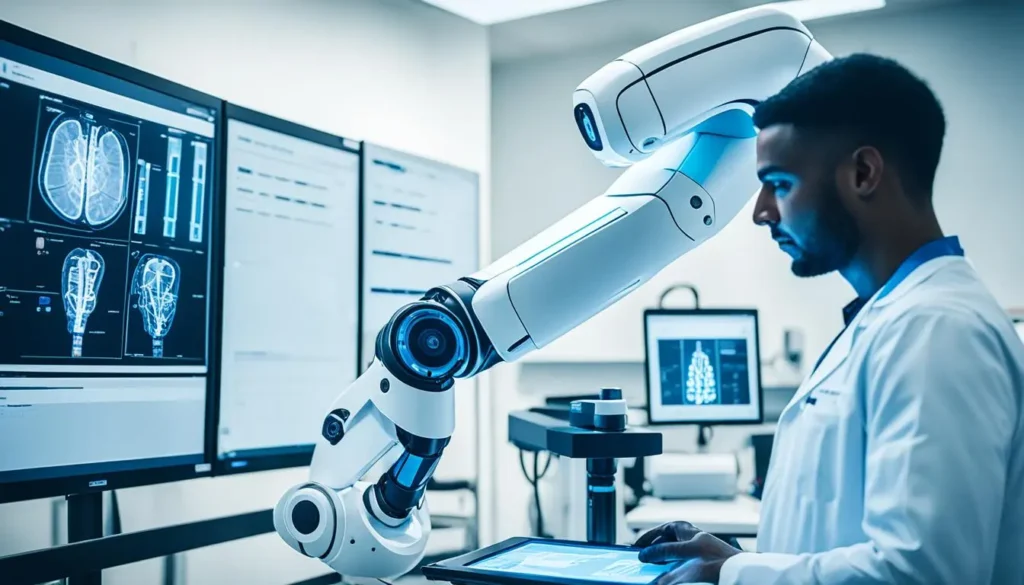
AI in Quality Assurance is becoming increasingly vital as we approach a projected shortage of 18 million healthcare workers by 2030, including 5 million fewer doctors. This stark reality underscores the need to transform healthcare delivery and leverage new technologies like AI. By ensuring high-quality, efficient, and equitable care, AI can play a crucial role in addressing these challenges.
AI’s potential to enhance healthcare systems aligns perfectly with the “quadruple aim“: improving overall health, enhancing the patient experience, boosting caregiver satisfaction, and reducing costs.
With the advent of diverse data sources and advancements in technology, including mobile devices and the Internet of Things (IoT), AI’s application in quality assurance is poised to revolutionize healthcare. It can make care more accurate, efficient, and patient-centered, driving the industry toward a better future.
Table of Contents
Key Takeaways
- AI-powered quality assurance can help healthcare systems address significant challenges in achieving the “quadruple aim” for healthcare.
- The convergence of multi-modal data and technology innovations in healthcare create opportunities to transform models of healthcare delivery through AI-augmented systems.
- Integration of AI into quality assurance processes can drive improvements in accuracy, efficiency, and patient-centeredness.
- AI-powered testing tools enhance accuracy, reliability, and efficiency, transitioning from manual to automated testing.
- AI-driven QA tools enable predictive analysis to forecast evolving user needs and behaviors.
AI Applications in Healthcare: Recent Breakthroughs
The healthcare industry has seen a big leap forward with Artificial Intelligence (AI). This includes machine learning (ML) and deep learning (DL). These technologies are changing how healthcare works, from making accurate diagnoses to planning treatments just for you.
Machine Learning and Deep Learning in Healthcare
Machine learning lets computer programs get better on their own. Deep learning is a type of algorithm that learns by looking at lots of examples. These AI tools are super helpful in healthcare. They help with medical diagnosis, disease prediction, patient monitoring, and drug discovery.
Using AI and ML in healthcare makes diagnosing diseases more accurate. It also cuts down the time it takes to get test results. AI can predict mistakes, help with making treatment plans, and give treatments that fit each patient better. For example, AI can look at medical images like MRIs and X-rays better than doctors, making diagnoses faster and more accurate.
Challenges in Adopting AI in Clinical Practice
Even though AI in healthcare has been a big focus for a decade, it’s still not widely used in clinics. Many AI tools for healthcare are still being made. Healthcare groups are trying to fit AI into their work without thinking about how it fits with their current ways, like how doctors work, what patients need, and safety and ethics.
Key AI Applications in Healthcare | Benefits |
---|---|
Predictive Analytics | Early detection of health problems, optimized treatment planning |
Medical Imaging Analysis | Faster and more accurate diagnoses, reduced errors |
Personalized Medicine | Tailored therapies based on genetics, lifestyle, and medical history |
Drug Discovery | Accelerated development, better patient outcomes |
Virtual Health Assistants | 24-hour care and support for chronic conditions |
Robotic Surgery | Improved precision, fewer invasions, and faster recovery |
Healthcare groups are facing challenges in using AI, but the benefits are huge. These technologies can improve patient care, make things run smoother, and help doctors make better decisions. The future of healthcare is closely tied to AI and how it’s used in a responsible way.
Building Effective and Reliable AI Systems
Creating strong and trustworthy AI for healthcare needs a focus on people. It means working together with a mix of experts like computer scientists, social scientists, and healthcare professionals. This team works together to make sure the AI meets real healthcare needs and fits into daily work.
Stakeholder Engagement and Co-creation
Starting AI development in healthcare means really understanding the clinical world. It’s important to study how healthcare places work and what users need and face. This way, AI and healthcare can work together to create solutions that fit right into clinical life.
Human-centered AI Approach
A human-centered AI method puts the focus on what healthcare workers and patients need. It’s about making AI systems that help and support, not replace, doctors and nurses. This means making sure the AI is easy to use, explains its decisions, and doesn’t show bias, ensuring everyone gets fair care.
“Effective AI development for healthcare begins with a deep understanding of the clinical context. An ethnographic approach to studying healthcare organizations, their operations, and the needs and constraints of end-users is essential.”
Adding AI to healthcare is a careful process. It’s about making sure the technology improves quality and care while keeping human control. With a focus on teamwork and people, healthcare can create AI systems that offer top-notch, fair, and patient-focused care.
Experimentation and Iterative Development
In the fast-changing world of healthcare tech, using Artificial Intelligence (AI) needs a careful, step-by-step plan. AI experimentation healthcare and iterative development AI are key to making AI systems that work well and are reliable.
Trying out AI tools is vital to see how they work, what they’re for, and their effects. With agile methodologies AI, health groups can quickly learn and tweak their plans based on feedback. This way, they can test many ideas at once, see what succeeds, and why.
AI Testing Approach | Benefits |
---|---|
Unit Testing | Verifies individual AI components’ functionality |
Integration Testing | Ensures seamless interaction between AI modules |
System Testing | Evaluates the AI system’s overall performance |
User Acceptance Testing | Validates the AI’s alignment with user requirements |
The AI testing protocols used in testing show the possible risks and ethical issues of the AI, like data privacy and security. This way, health groups can make their AI better, making sure it meets their needs and helps patients.
“AI-driven test automation in quality assurance reshapes the industry, enabling businesses to deliver error-free software products to customers.”
By using AI experimentation healthcare and iterative development AI, health groups can handle the challenges of adding AI, build trust, and create new solutions that improve patient care and results.
AI in Quality Assurance: Ensuring High Standards
Regulatory Compliance and Safety Standards
AI is becoming more common in healthcare, so it’s vital to follow safety rules and standards. Luckie Health is a top healthcare marketing agency in North America. They focus on being open, responsible, and fair in their AI testing. This builds trust and integrity in checking quality.
The Institute of Medicine (IOM) has six quality dimensions for AI in healthcare. Safety is the main goal, aiming for zero harm. Following rules like FDA, CMS, ISO 13485, and IEC 62304 is key. It’s also important to check AI systems, investigate incidents, and improve processes safely.
Ongoing Evaluation and Safety Culture
Being able to understand and see how AI works is crucial for safe use in healthcare. Luckie Health’s Reflect platform uses natural language processing. It helps testers make test scenarios sound like real human commands. This shows the need for a human-focused approach in making and using AI.
Luckie Health combines industry knowledge with AI to lead in healthcare tech and digital solutions. As AI becomes more important in checking quality, focusing on ethical and safe practices is key. This ensures healthcare services are reliable, trustworthy, and of high quality.
“AI does not replace human testers but complements their cognitive skills, creativity, problem-solving abilities, and emotional intelligence. Human testers provide irreplaceable understanding and contextual decision-making, offering insights into user experience that AI cannot replicate.”
Metric | Value |
---|---|
Projected Market Value of AI in Healthcare QA | USD 4.0 billion by 2026 |
Projected AI Testing Adoption in Development Projects | 50% by 2026 |
Typical Manual QA Coverage of Customer Interactions | 2 to 5% |
AI-powered QA Coverage of Customer Interactions | 100% |
AI for Effective and Patient-Centered Care
AI is changing healthcare for the better, making it more effective and focused on each patient’s needs. It uses advanced analytics and machine learning to offer services that fit each person’s unique situation. This approach makes healthcare more personal and efficient.
Testing and Validation in Real-World Settings
To make sure AI in healthcare works well, it needs to be tested and validated in real clinics. It’s important to check if AI is really helping patients. By doing this, we can see what works and what doesn’t, making healthcare better for everyone.
Data Quality, Management, and Informed Consent
Good data is key for AI in healthcare. Keeping data safe and private is crucial for trustworthy results. It’s also important to get patients’ okay before using AI in their care. By focusing on data quality and talking openly with patients, we can build trust and make AI a real help to patients.
Key Benefits of AI in Patient-Centered Care | Potential Challenges |
---|---|
Personalized treatment recommendations based on individual patient data Improved clinical decision-making and reduced medical errors Enhanced patient engagement and satisfaction through AI-powered tools Optimized resource allocation and reduced administrative burden for healthcare providers | Ensuring high-quality, unbiased data for AI models Gaining patient trust and addressing ethical concerns around AI use Integrating AI solutions seamlessly into existing clinical workflows Continuously monitoring and improving AI performance in real-world settings |
By tackling these issues and focusing on patients, healthcare can fully benefit from AI effective healthcare. This leads to better care, happier patients, and helps doctors do their best work.
AI in Quality Assurance: Timely and Efficient Care
AI is changing healthcare, making it better and faster. It helps doctors work smarter, not harder. This means they can focus more on helping patients and less on paperwork.
Optimizing Clinician Time and Reducing Administrative Burden
AI is making healthcare work flow better. It lets doctors focus on what they do best: diagnose and treat patients. AI timely healthcare tools look at patient data, do paperwork for them, and give insights in real time. This means doctors can spend more time with patients.
AI efficient healthcare also cuts down on paperwork for doctors. This lets them talk more with patients. By making doctors’ work more efficient, AI optimizing clinician time and AI reducing administrative burden make healthcare better for everyone.
Key Performance Indicator (KPI) | Metric |
---|---|
Diagnosis timeline | Reduced by 20% on average |
Treatment planning timeline | Decreased by 15% |
Administrative time reduction | 50% decrease in clinician time spent on non-clinical tasks |
Keeping an eye on how AI works is key to making sure it keeps helping healthcare. We need to check in often and make changes as needed. This way, AI stays a big help in giving timely and efficient care.
“AI-driven predictive analytics in healthcare can optimize processes, reduce errors, and identify high-risk patients for early intervention, leading to lowered healthcare costs and increased operational efficiency.”
AI in Quality Assurance: Ensuring Equitable Care
The healthcare industry is now using AI more often. It’s important to make sure care is fair for everyone. We must check AI systems for bias to improve health equity and patient safety. It’s key to reduce bias in AI to stop unequal healthcare access for some groups.
Addressing Bias and Fairness in AI Systems
Working on bias and fairness in AI is ongoing. Healthcare groups need to be careful when adding AI to their work. They should focus on being open, taking responsibility, and keeping an eye on AI to avoid bias and unfairness.
- Check AI tools well for quality, safety, reliability, and fairness before using them.
- Follow rules for using AI responsibly, like keeping data private and being clear about how it works.
- Work with lawmakers to test AI on a big scale and make sure it’s safe and fair.
- Make sure AI learns from data that shows everyone equally, not just some groups.
By focusing on fair care and fixing AI bias, healthcare can use AI better. This way, everyone gets the same chance to get good healthcare.
Bias Type | Description | Impact on Healthcare |
---|---|---|
Algorithmic Bias | Bias comes from how AI is made and trained, often from bad or incomplete data. | Can cause wrong or unfair diagnoses and treatment plans. |
Historical Bias | Bias in old healthcare data shows deep-seated unfairness and unequal access. | Keeps health gaps and makes AI healthcare decisions biased. |
Human Bias | Bias comes from people making AI, like doctors or those who decide on AI use. | Can cause unfair treatment and make people doubt AI in healthcare. |
“Kaiser Permanente uses a careful AI approach. They check tools for quality, safety, reliability, and fairness before adding them.”
AI in Quality Assurance: Empowering Clinical Judgment
In healthcare, AI has a big role in making sure things are done right. But, it’s important to remember that AI should help, not take over from doctors. This keeps patients safe and builds trust. AI tools in healthcare must follow medical guidelines. This lets doctors use their skills and make good decisions.
AI systems should augment and empower doctors, not replace them. With AI tools, doctors can get better at diagnosing, work more efficiently, and make smarter choices. This AI-clinician collaboration creates a strong partnership. Both sides work together to make the best AI-augmented clinical decisions.
It’s also key to check how well AI training works and how well technology is used. Doctors need good training and support to use AI well in their work. This helps them use AI to its fullest potential while keeping their professional skills and freedom.
“AI should not replace clinicians, but rather empower them to make more informed decisions and deliver better patient care.”
By finding the right balance between AI and doctor’s judgment, healthcare can offer top-quality care. This care focuses on the patient and follows safety, trust, and ethical standards.
Best Practices in AI Development and Deployment
Creating and using AI in healthcare needs a careful plan. It should include the best ways to do things. Using agile methods, thorough testing, and checking each other’s work is key. This makes sure AI in healthcare is safe, works well, and is reliable.
Adopting agile methods is a top practice. Agile helps teams work together, change quickly, and keep improving. It’s vital in healthcare where things are always changing. Teams can quickly adjust to new needs, get feedback, and update their AI models and solutions.
Testing AI solutions carefully is also crucial. This means setting aside data for training, checking, and testing. It’s important to look at things like how accurate the AI is and how well it works. Using special tests like k-fold cross-validation makes the testing stronger.
Best Practices | Key Benefits |
---|---|
Agile Methodologies | Flexibility, Collaboration, Iterative Improvement |
Rigorous Testing Protocols | Ensuring Safety, Effectiveness, and Reliability |
Peer Reviews | Identifying Potential Biases and Ethical Concerns |
Peer reviews are vital too. They spot biases, ethical issues, and ways to get better. By getting feedback from different people, like doctors, data experts, and ethicists, teams can make their AI better.
Keeping up with changes in tech and healthcare is a must. Following rules and guidelines helps small companies and startups. It makes sure their AI is safe and works well, even if it’s not required by law.
By following these best practices, healthcare groups can make and use AI that’s top quality and safe. This builds trust with patients and healthcare workers.
Conclusion
The healthcare industry is changing fast with the help of artificial intelligence (AI). It’s crucial to make sure care is safe, high-quality, and fair for everyone. We need strong quality checks that look at how safe, effective, fair, and easy to get AI tools are.
The Institute of Medicine talks about six key areas for quality: safety, effectiveness, patient focus, on time care, efficiency, and fairness. Using these ideas helps create strong quality checks for AI in healthcare. This way, we can make sure AI helps improve patient care and overall healthcare quality.
It’s important to keep updating these quality checks as technology and healthcare change. This makes sure AI in healthcare stays top-notch, brings new ideas, works better, and helps everyone get fair care. With a focus on people and smart use of AI, healthcare leaders can make the most of this new technology. This leads to the best care for patients and their communities.
FAQ
What is the potential of artificial intelligence (AI) in transforming healthcare quality assurance?
AI could change how we practice medicine and deliver healthcare. It can help healthcare systems worldwide tackle big challenges. These include improving health, enhancing patient care, making caregivers happier, and cutting costs.
What are the key AI subfields that are relevant to healthcare applications?
AI includes subfields like machine learning (ML) and deep learning (DL). These add smarts to healthcare tools. ML lets computers get better on their own. DL is a type of ML that learns by looking at lots of examples.
What are the challenges in adopting AI in healthcare?
Adopting AI in healthcare faces hurdles. One big issue is forcing AI into healthcare without thinking about local needs. This includes clinical workflows, user needs, trust, safety, and ethical issues.
What is a human-centered approach to building effective and reliable AI-augmented healthcare systems?
A human-centered AI approach means understanding healthcare systems deeply. It focuses on the main problems and needs in healthcare. It also looks at how AI can fit into clinical settings.
How can experimentation and feedback help in the development of AI systems for healthcare?
Trying out new ideas and seeing what works is key. This helps learn what’s effective and why. Feedback and experimentation are vital for making AI systems better. They help figure out the AI’s purpose and its impact on users and data privacy.
How can the six dimensions of quality described by the Institute of Medicine (IOM) be applied to AI in healthcare quality assurance?
The six quality dimensions from the Institute of Medicine (IOM) help design AI quality frameworks. These dimensions are safety, effectiveness, patient focus, timeliness, efficiency, and fairness.
What are the key considerations for ensuring the effectiveness and patient-centricity of AI in healthcare?
Making AI effective and patient-focused needs thorough testing in real settings. It’s important to focus on data quality and getting patient consent. Regular checks and reporting are key to see if AI improves care.
How can AI optimize clinicians’ time and improve healthcare delivery?
AI can make doctors’ work more efficient and speed up diagnosis and treatment. It’s important to set clear goals and keep improving. AI should make work easier, not harder, by streamlining tasks and improving how different systems talk to each other.
How can AI-powered quality assurance ensure equitable care?
Checking AI for bias is crucial to ensure fair healthcare. It’s important to reduce AI bias to prevent unequal healthcare access and outcomes. Making AI fair should be an ongoing effort in healthcare.
How should AI be designed to complement and empower clinicians?
AI should support and empower doctors, not replace them. This ensures safety and keeps patient trust. AI should follow medical guidelines, letting doctors use their skills and judgment.
What are the best practices in the development and deployment of AI solutions for healthcare?
Using best practices like agile methods and thorough testing is key for AI in healthcare. Keeping up with changes and following rules and guidelines is important. This helps ensure AI is safe and effective.
1 comment
Thanks I have recently been looking for info about this subject for a while and yours is the greatest I have discovered so far However what in regards to the bottom line Are you certain in regards to the supply