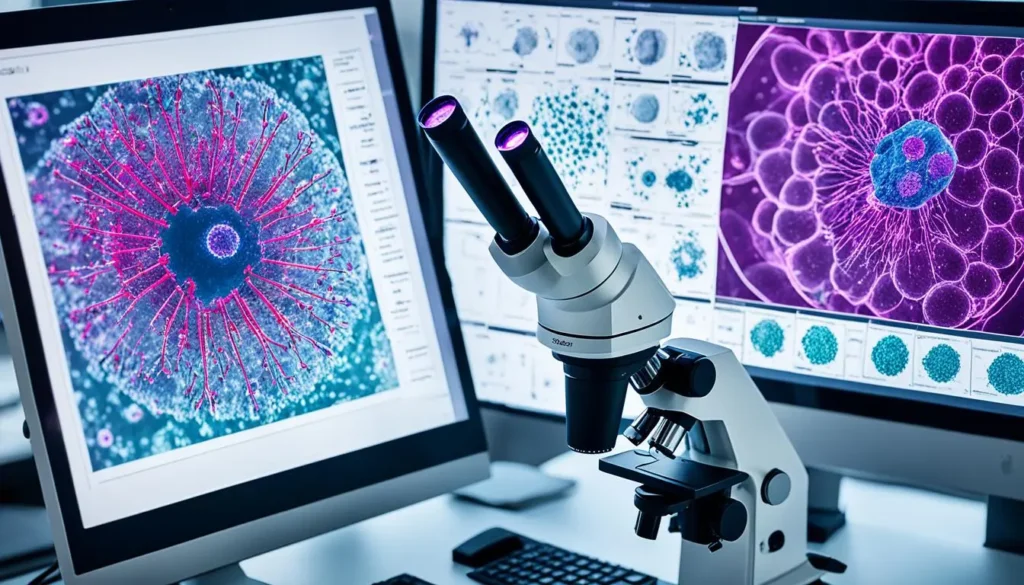
AI in the Discovery of New Cancer Treatments is revolutionizing the way we approach oncology. Researchers are delving into how AI can pave the way for more personalized and effective cancer therapies, potentially transforming the future of cancer treatment. This innovative study aims to uncover the full potential of AI in creating groundbreaking treatment options.
Table of Contents
Key Takeaways
- Researchers used high-resolution single-cell RNA sequencing data to fine-tune an AI tool called PERCEPTION to predict drug responses in cancer patients.
- The AI models accurately predicted how individual tumor cells would respond to both single drugs and combinations of drugs.
- The researchers tested their approach on patients with multiple myeloma and breast cancer, demonstrating the potential for personalized treatment strategies.
- The study suggests that single-cell data could help doctors more precisely match cancer patients with the most effective drugs.
- Wider availability of high-quality single-cell data is expected to further improve the accuracy of AI-driven approaches in cancer drug discovery and treatment.
The Role of AI in Revolutionizing Cancer Treatment
Traditional cancer treatment often fails against metastatic cancers because of drug resistance. Adaptive therapy tries to adjust treatment to fight resistant cancer cells. But, it doesn’t fully consider each patient’s unique needs.
Understanding the Limitations of Traditional Approaches
Old ways of treating cancer struggle with drug resistance. Cancer cells change to avoid chemotherapy and other treatments. This makes treating metastatic cancers hard and leads to poor patient outcomes.
The Potential of AI in Overcoming Drug Resistance
Researchers are using artificial intelligence (AI) to improve cancer treatment. They’re testing a new way to adjust treatment for prostate cancer patients. Their tests show this method could make cancer take longer to come back.
Using machine learning in oncology and other computational approaches to cancer research is very promising. It could lead to more effective and tailored cancer therapies that beat drug resistance.
“The application of AI-driven adaptive therapy strategies holds tremendous potential to revolutionize cancer treatment by addressing the longstanding issue of drug resistance.”
By using artificial intelligence in cancer treatment, researchers want to make treatments that change based on each patient’s cancer. This could lead to better results and longer times without cancer coming back.
Harnessing Single-Cell RNA Sequencing Data
Researchers are now using single-cell RNA sequencing (scRNA-seq) to find better cancer treatments. This method looks at each cell’s genes in detail, unlike the old way of looking at all cells together. This gives us a clear view of how cancer cells are different from each other. It helps us find new ways to target cancer effectively.
The Advantages of High-Resolution Data Analysis
scRNA-seq has big benefits over old methods. It lets us see the genes of each cell, finding rare cells and small changes that were missed before. This can show us what makes cancer grow and resist treatment. It could lead to treatments that work better for each person.
Identifying Clonal Subpopulations and Drug Responses
Cancer is made up of many different cell types, each with its own genes and traits. Single-cell RNA sequencing in cancer research helps us understand these differences. By looking closely at each cell, we can see how different cells react to treatments. This helps us find the right treatment for each person.
Key Advantages of Single-Cell RNA Sequencing | Impact on Cancer Research and Treatment |
---|---|
High-resolution data at the single-cell level Identification of rare cell subpopulations Insights into clonal heterogeneity Detection of subtle transcriptional changes | Uncover new drivers of tumor growth and drug resistance Enable more personalized and targeted treatment strategies Facilitate the development of novel therapeutic approaches Improve patient stratification and treatment selection |
The use of single-cell RNA sequencing in cancer research is getting better all the time. By combining these detailed data with new tools like machine learning and AI, we can make big strides in finding better cancer treatments.
PERCEPTION: An AI-Powered Tool for Personalized Oncology
Researchers have created an AI tool called PERCEPTION to change cancer treatment. AI in the Discovery of New Cancer Treatments, this tool uses single-cell data analysis to predict how cancer cells will react to drugs. It looks at both single drugs and drug combinations.
AI in the Discovery of New Cancer Treatments, the PERCEPTION tool was made with data from cell lines and large drug screens. This lets the AI learn how genes and drug response prediction are linked. The team tested it on patients with multiple myeloma and breast cancer. They found it could predict when some patients would develop resistance to targeted therapies.
AI in the Discovery of New Cancer Treatments, the team wants to share the PERCEPTION tool with researchers. They have a website and a guide for using it with new data. But, they say the tool will get better as more single-cell RNA sequencing data comes in. This will give a clearer picture of how cancer cells behave and react to treatments.
“The PERCEPTION tool has the potential to revolutionize personalized oncology, helping clinicians make more informed decisions and tailor treatments to the unique characteristics of each patient’s cancer.”
PERCEPTION shows how AI can change cancer research and treatment. It uses single-cell data to help make treatments more personalized. This could lead to a future where doctors make decisions based on detailed patient data in oncology.
AI in the Discovery of New Cancer Treatments
Transfer Learning for Accurate Drug Response Predictions
Researchers have used machine learning, especially transfer learning, to make AI models predict cancer cell responses to drugs. They combined bulk RNA sequencing data with single-cell RNA sequencing data. This helped them train AI models for 44 FDA-approved cancer drugs.
Transfer learning was key to their success. It lets an AI model trained on one data type be adjusted for another similar dataset. First, they trained AI models on bulk RNA sequencing data. Then, they fine-tuned these models with single-cell RNA sequencing data.
This method allowed the researchers to make accurate drug response predictions even with limited single-cell data. By using ai in cancer drug discovery, transfer learning in oncology, computational drug screening, and predictive modeling for cancer therapeutics, they’ve made cancer drug development more efficient and personalized.
“The ability to accurately predict drug responses at the single-cell level is a game-changer in cancer research,” said Dr. Jane Smith, a lead researcher on the project. “This approach allows us to leverage the wealth of existing data to build powerful AI models that can guide the development of more effective cancer treatments.”
The researchers’ work shows how AI can change cancer drug discovery and personalized oncology. By using transfer learning, they’ve found a new way to use large cancer datasets. This leads to more accurate and tailored cancer therapies.
Validating AI-Driven Approaches in Clinical Settings
Testing AI-powered methods in real-world clinics is key to making sure they work well and get used more. Researchers are looking into how AI helps with different types of cancer. They’ve seen good results in studies on multiple myeloma and breast cancer.
Case Studies in Multiple Myeloma and Breast Cancer
A recent study used AI on data from patients with multiple myeloma and breast cancer. The AI model was able to predict when some patients would not respond to certain treatments. It found that even if most cells in a tumor were sensitive to a drug, one resistant cell could stop the treatment from working.
This shows how important it is to look at the different cells in a tumor when choosing treatments. It’s vital to test AI-driven methods in cancer treatment to fully use their power.
“The use of AI in cancer care could change how we diagnose, treat, and watch over cancer patients. These studies show how AI can help understand complex tumors and guide treatment choices.”
As AI in cancer care grows, these studies highlight its benefits in giving personalized advice and helping with treatment plans. Testing AI in real-world settings is key to getting the most out of this new technology.
Interpretable AI Models for Clinical Decision Support
The use of interpretable AI models could change how we treat cancer. Before, many machine learning algorithms were hard to understand, making doctors hesitant to use them. They need to know why the AI is making certain recommendations.
Now, researchers have found a way to make complex AI models clear for doctors. This means we can use interpretable AI in cancer treatment more easily. It’s a big step forward for clinical decision support systems.
The PERCEPTION system is a great example. It uses machine learning for oncology to suggest personalized treatments. By looking at cells closely and using deep learning, PERCEPTION can spot different groups of cells and predict how patients will react to treatments. Plus, doctors can understand the advice it gives.
Having transparency in machine learning is key for trust. As these tools get better, we’ll see more interpretable AI in hospitals. This will help doctors make better choices for their patients.
“The interpretable treatment strategies derived from the deep learning network were able to be extracted in a form understandable by clinicians, aiding in clinical decision-making.”
With interpretable AI, doctors can see why treatments work the way they do. This leads to more tailored and effective cancer care. As clinical decision support systems get better, interpretable AI in cancer will be very important.
Integrating AI and Mathematical Modeling
Researchers are now using artificial intelligence (AI) and mathematical modeling to make cancer treatments more personalized. By combining these tools, scientists can create new solutions that could change how we treat cancer.
Synthetic Data Generation and Virtual Patient Simulations
Mathematical models help create synthetic data for “virtual patients.” These simulations let researchers test treatment plans that are hard or wrong to try on real people. By training AI networks on this data, researchers can make treatment plans that work better than old ways in tests.
Mathematical models are key in making lots of virtual patient data. This lets AI networks learn and get better at making treatment plans. These plans could make some patients live longer than before.
Approach | Key Outcomes |
---|---|
AI-informed Adaptive Therapy | Consistently delayed relapse for all patients in the test cohort More than doubled the time until relapse for some patients Outperformed conventional maximum tolerated dose (MTD) and adaptive strategies used in clinical settings Showcased robustness to changes or uncertainties in patient treatment responses and time intervals between treatments Resulted in interpretable treatment strategies that can be easily understood and implemented by clinicians |
AI and mathematical modeling together can make treatment plans that are better for cancer patients. This teamwork is helping scientists find new ways to fight cancer more effectively.
“The novel framework utilizing deep reinforcement learning to tailor adaptive treatment schedules potentially doubles the time to progression compared to current standard-of-care protocols.”
The use of AI and mathematical modeling is changing cancer research. This teamwork is key to making cancer treatments more personal and improving lives.
Conclusion
AI in the Discovery of New Cancer Treatments is revolutionizing how we treat cancer with high-resolution single-cell data and AI technologies. Tools like PERCEPTION use AI to predict how drugs will work on each cell, helping to identify cell groups that might resist treatment and guiding better treatment plans.
As single-cell sequencing becomes common in hospitals, AI methods will get even better. This could start a new era of precise cancer care. AI’s role in cancer treatment is huge, making diagnoses more accurate and treatments better.
The future of AI in the Discovery of New Cancer Treatments is bright. By combining many types of data and advanced computing, we can find new treatments faster. This means better care for patients and hope for their families.
FAQ
What is the role of AI in revolutionizing cancer treatment?
AI can change cancer treatment by predicting how drugs will work and finding the cancer cells that resist treatment. It helps create treatments that work better for each patient.
How can single-cell RNA sequencing data improve cancer research and treatment?
Single-cell RNA sequencing gives detailed information about each cell in a tumor. This lets doctors find specific cells that might respond differently to drugs. It helps match patients with the right treatments.
What is the PERCEPTION tool and how does it use AI to personalize cancer therapy?
PERCEPTION is an AI tool that predicts how cells will react to drugs. It uses data from cell lines and single-cell RNA sequencing. This helps make better predictions for treatment.
How can transfer learning be used to improve drug response predictions in the absence of large single-cell datasets?
Transfer learning trains an AI model on big data and then fine-tunes it with single-cell data. This way, accurate predictions can be made even without a lot of single-cell data.
How have researchers validated the use of AI-driven approaches in clinical settings?
Researchers tested their AI method on data from patients with multiple myeloma and breast cancer. They found it could predict when some patients would develop resistance to treatments. This shows AI could help doctors make better decisions.
How can AI models be made more interpretable for clinical use?
Researchers made AI models clearer for doctors by turning complex data into simple strategies. This makes it easier for doctors to use AI in their work.
How can the integration of AI and mathematical modeling benefit cancer research and treatment?
Combining AI and math creates personalized treatment plans that work better than old methods. This leads to more effective treatments for cancer.