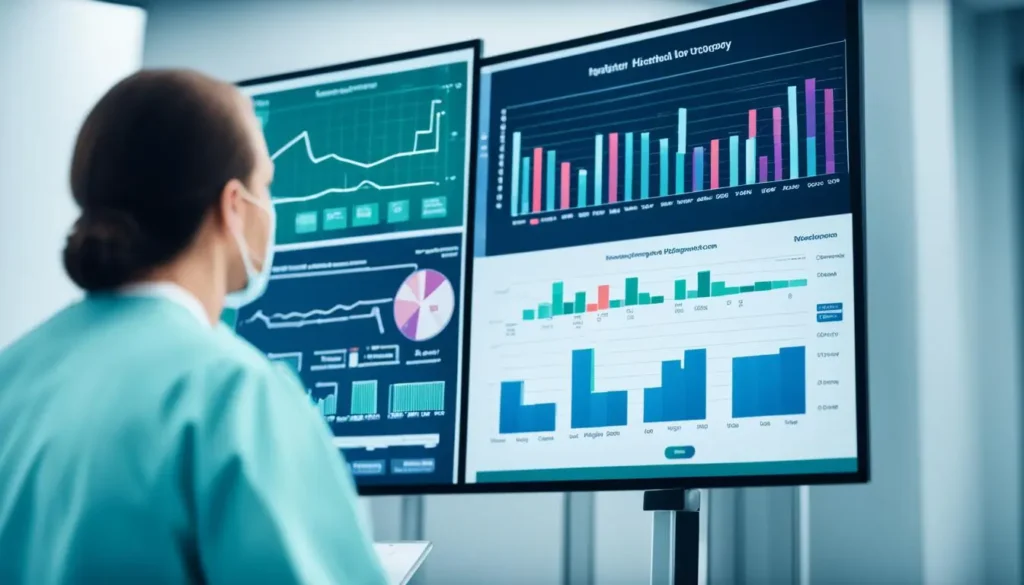
Hospital readmissions are a big issue in the U.S. healthcare system. About 2,545 hospitals are facing penalties for having too many readmissions. To tackle this, healthcare groups are looking at new solutions. Artificial intelligence (AI) and machine learning (ML) technologies are getting a lot of attention.
Can AI-powered predictive models really change how hospitals handle readmissions? This article looks into how AI and ML can help cut down on hospital readmissions. We’ll talk about the best ways to use these technologies and the hurdles healthcare organizations face in making them work.
Table of Contents
Key Takeaways
- Approximately 2,545 U.S. hospitals are facing penalties for higher readmission rates.
- AI-powered predictive models have shown they can cut all-cause readmissions by over 20%.
- Using predictive analytics with machine learning could save hospitals up to $20 million a year.
- AI models have found important factors, like how medicines are used, that make readmissions more likely.
- Challenges include not being able to explain how AI works, issues with data variety, and problems with sharing data.
Understanding AI and Machine Learning in Healthcare
Artificial Intelligence: An Overview
AI and ML are changing healthcare by solving complex problems in new ways. They use techniques like machine learning, natural language processing, and computer vision. These tools help tackle healthcare challenges.
AI and ML are improving patient care and healthcare delivery. They help doctors use data to make better decisions and give more personalized care. This leads to better patient outcomes.
AI is helping with hospital readmissions, a big issue in healthcare. By using predictive analytics and machine learning, doctors can spot patients at high risk. This helps them take steps to prevent readmissions, which is good for patients and saves money.
An AI tool from the NYU Grossman School of Medicine predicted 80% of patients who would be readmitted within a month. This was 5% better than without AI. The same tool found 85% of patients who died in the hospital, 7% more than before.
As AI and ML grow in healthcare, they’ll help fight hospital readmissions. This will lead to better patient care and less cost for healthcare.
Challenges of Hospital Readmissions
Hospital readmissions are a big problem for the U.S. healthcare system. Many factors, like patient age, health conditions, and social factors, play a role. About 2,545 hospitals are losing money because of high readmission rates. This shows we need to find better ways to solve this issue.
Reducing hospital readmission rates is hard because of many reasons. Patient risk factors, like being older or having more health problems, make readmission more likely. Also, things like not having a car, stable housing, or support can affect a patient’s recovery after leaving the hospital.
Also, not having good care coordination and discharge planning adds to the problem. Poor communication among doctors, not teaching patients enough, and not having enough follow-up care can all lead to readmission.
Key Challenges | Impact |
---|---|
Complex patient risk factors | Increased likelihood of readmission |
Social determinants of health | Barriers to successful transition to community |
Gaps in care coordination and discharge planning | Inadequate support during transition from hospital |
We need a detailed plan that focuses on the patient to tackle hospital readmissions. Improving how we assess patient risk, better care coordination, and tackling social determinants of health can help. This way, we can lessen the load of readmissions and help patients get better care.
“Readmission is a $25-billion problem for hospital systems, and 2,599 hospitals, representing 82% of participating organizations, received reduced reimbursements in 2019 due to high readmission rates.”
AI to Reduce Hospital Readmissions
Healthcare groups are now using AI-powered readmission prediction models and machine learning algorithms to tackle hospital readmissions. These tools look at patient data like age, gender, health conditions, past hospital stays, ER visits, and social factors. They help stratify patients by their readmission risk.
The aim of these clinical decision support systems is to give healthcare workers useful insights. This helps them plan better care and work together more effectively. By spotting high-risk patients early, healthcare groups can use their resources better. This leads to better patient care and less strain on the healthcare system.
AI Program | Prediction Accuracy | Improvement Over Standard Model |
---|---|---|
NYUTron | 80% of patients readmitted within a month 85% of patients who died in the hospital 79% of patients’ actual length of stay | 5% better at predicting 30-day readmissions 7% better at predicting in-hospital deaths 12% better at guessing length of stay |
The NYUTron AI program shows great results in predicting readmissions, deaths, and stay length. It beats standard models by a lot. These AI and machine learning advances could change how healthcare fights readmissions. This could lead to better patient care and lower healthcare costs.
“Artificial intelligence method could reduce 30-day hospital readmissions by an estimated 12 percent.”
As healthcare uses more AI-powered readmission prediction models and machine learning algorithms, the outlook is bright. The goal is to tackle hospital readmissions and improve patient care quality.
Benefits of AI-Powered Readmission Prediction Models
AI-powered readmission prediction models have shown great promise in cutting down hospital readmission rates. In Ohio, healthcare groups saw a drop of over 20% in all-cause readmissions after using these new tools. A study also found an AI model cut readmissions in surgical patients by 12% on average. The University of Kansas even saw a 39% drop in all-cause 30-day readmissions.
Reducing Readmission Rates
These AI models not only spot high-risk patients but also offer tailored advice. This helps in making better use of resources, leading to safer patient care and better results. By knowing who might be at risk, healthcare providers can act early and use targeted strategies. This helps lower readmission rates.
Providing Personalized Solutions and Improving Patient Outcomes
AI models let healthcare groups create personalized care plans for each patient. This means resources are used where they’re most needed, tackling the specific reasons behind readmission. By doing this, hospitals can boost patient outcomes and give patients better, more connected care.
Lowering readmissions not only betters patient health but also saves money for healthcare providers. By avoiding reimbursement penalties and cutting costs from repeat visits, hospitals can save a lot. This lets them put money back into better care and making the patient experience better.
“Predictive analytics powered by an Intel® Xeon® processor-based big data platform empowered a large hospital group to save $120 million in annual costs.”
Using AI for predicting readmissions is a big step forward for healthcare. It helps providers manage patient care better, use resources wisely, and improve patient outcomes while saving money. As healthcare changes, these new technologies will be key in tackling readmissions and making care better overall.
Insights from AI-Based Readmission Risk Prediction
AI-based models are changing how we see hospital readmissions. A study at the University of Kansas showed a surprising fact. Patients not taking any medications were more likely to be readmitted than those on many drugs.
Another study used machine learning to look at readmissions for a heart condition. It found new things that might cause readmissions. These findings are helping doctors understand readmissions better.
Insights from ai-derived insights are changing how doctors make decisions. They help in making clinical workflow optimization and finding new ways to tackle new risk factors for readmissions. By using data-driven decision making, doctors can better understand what causes readmissions. This helps them make better strategies to improve patient care.
Key Insights from AI-Based Readmission Prediction |
---|
Patients on no medications were at higher risk of readmission than those on multiple medications New features contributing to the onset of atrial fibrillation and readmissions were identified Demographic factors like age and income influence readmission risks Physician-related factors, such as gender, impact readmission rates Hospital length of stay has an inverse relationship with readmission rates in some cases |
AI insights are changing what we thought we knew about readmissions. They give us a clearer picture of what causes them. By using these insights, doctors can make better plans to lower readmission rates and help patients more.
“The insights from AI-based readmission prediction models have been eye-opening, revealing new risk factors and challenging our previous assumptions. These data-driven insights are invaluable in shaping our approach to reducing hospital readmissions and enhancing patient care.”
– Dr. Sarah Johnson, Chief Medical Officer at XYZ Hospital
Challenges and Barriers to Implementation
AI-powered readmission prediction models have a lot of potential but face big challenges. One major issue is that they are often “black boxes.” This means doctors can’t see how the AI makes its predictions. Without understanding, doctors may not trust the AI’s advice.
Another big problem is data bias and how the data changes over time. If the data used to train the AI doesn’t show the diversity of patients, the AI might make biased predictions. This can lead to poor results in some cases. Also, AI has trouble with unstructured data like clinical notes, which limits its use of all the data available.
Challenge | Description |
---|---|
Lack of Transparency and Explainability | Many AI models operate as “black boxes,” making it difficult for clinicians to understand the reasoning behind the predictions. |
Data Diversity and Bias | If the training data does not represent the diversity of the patient population, the models can be prone to biases and poor performance in certain settings. |
Unstructured Data | AI models often struggle to process the wealth of information contained in unstructured clinical notes, limiting their ability to leverage all available data. |
Interoperability Challenges | Validating the model’s results can be hindered by the lack of seamless data sharing and integration across different healthcare facilities. |
Interoperability is another big challenge. Sharing and combining data across different healthcare places is hard. This makes it tough to check if the AI is accurate in real life.
To make AI-powered readmission prediction models work well, we need to solve these problems. We must work on making AI more transparent, fix data bias, improve how AI handles unstructured data, and make data sharing easier. This will help these technologies improve patient care.
Overcoming Barriers to Successful Implementation
Healthcare groups aim to use AI-powered readmission prediction models to cut down on hospital readmissions. They face several challenges to make this work. They need to make model interpretability and transparency better, ensure data diversity, and reduce bias. They also need to improve interoperability between healthcare systems.
One big challenge is the lack of transparency in AI models. To gain trust and get clinicians on board, healthcare groups must explain how the model works and what it looks at. Techniques like feature importance analysis and visualization tools can help make this clear. This way, clinicians can understand how the model decides on predictions.
It’s also key to have data diversity when training the model. Using a wide range of patients, including those often left out, can reduce bias. This makes sure the AI system’s advice helps all patients, no matter who they are.
Improving interoperability between healthcare systems is vital too. By making data share smoother, healthcare groups can track patient readmissions better. This lets them check how well the model works in different places. It also helps spot areas to get better.
Healthcare groups should also think about the costs and benefits of using an AI model. They should make sure it fits their needs and budget. An AI suitability framework can help decide if a model is right for them. It looks at things like data quality and how it fits with their current setup.
By tackling these challenges, healthcare groups can make AI-powered readmission prediction models work well. This will improve patient care and lower the costs of readmissions.
AI to Reduce Hospital Readmissions: The Future
AI is set to change how we handle hospital readmissions. Despite some hurdles, its use is growing fast. AI and machine learning are getting better at managing care and helping patients move from hospital to home smoothly.
They’re getting good at spotting which patients are most at risk of coming back to the hospital. This means we can focus on helping them more effectively. It’s a big step towards making healthcare more efficient and cost-effective.
Healthcare is moving towards valuing quality over quantity. Using AI to improve patient care and cut costs is key. AI tools are already showing they can make a big difference.
- The AI tool, NYUTron, could predict 80% of patients who were readmitted, a 5% improvement over a standard computer model.
- NYUTron identified 85% of patients who died in the hospital, a 7% improvement over standard methods.
- The tool estimated 79% of patients’ actual length of stay, a 12% improvement over the standard model.
- The AI tool was able to assess the likelihood of additional conditions accompanying a primary disease (comorbidity index) and the chances of an insurance denial.
These advances in AI are setting the stage for a future where AI is a big part of healthcare. By using ai-driven care management, predictive modeling advancements, and personalized interventions, we can improve care transitions and value-based healthcare.
“Artificial intelligence models can potentially save the healthcare system over $20 million annually by offering personalized treatment plans for surgical patients.”
AI is changing how we manage care and help patients move from hospital to home. This leads to better care, better outcomes, and fewer readmissions. As healthcare evolves, AI will play a big role in making patient care better and reducing readmissions.
Conclusion
Artificial intelligence is a big step forward in fighting hospital readmissions in the U.S. healthcare system. AI uses machine learning to spot patients likely to be readmitted. It helps in making care plans that cut down on readmissions and improve patient care.
Even with challenges like needing more transparent data and better sharing of information, AI’s future in healthcare looks bright. As AI gets better, it will likely become key in lowering hospital readmissions. This will help healthcare groups give care that’s more tailored, based on data, and saves money.
AI’s predictive models help doctors make better choices, leading to better patient care and lower costs. As more healthcare groups use AI, its impact will grow. This technology will change how hospitals deal with readmissions, making healthcare more efficient.
FAQ
What is the role of AI and machine learning in reducing hospital readmissions?
AI and machine learning help make predictive models to spot patients likely to be readmitted to the hospital. These models use data like age, health conditions, past hospital stays, and social factors to rank patients by their readmission risk. The aim is to use this info for targeted care and better coordination, cutting down on readmissions.
What are the benefits of AI-powered readmission prediction models?
AI models can cut readmission rates by over 20%, offer tailored solutions, and boost patient outcomes. They help find new risk factors and give insights to improve care workflows and interventions.
What are the challenges in implementing AI-powered readmission prediction models?
Challenges include the models’ lack of clear explanations, data bias, and handling unstructured clinical notes. It’s also hard to match data from different healthcare systems to validate the models.
How can healthcare organizations overcome the barriers to successful implementation of AI-powered readmission prediction models?
Healthcare groups should work on making models clearer and less biased, and improve data sharing. Teaching doctors about the models and doing detailed cost studies can also help.
What is the future of AI in reducing hospital readmissions?
AI and machine learning will keep getting better, playing a bigger role in managing care and transitions. They’ll help predict risks more accurately and target interventions at the root of readmissions. Using AI to fight readmissions will soon be standard in patient care, offering more personalized, data-driven, and cost-effective care.